Introduction
In today’s data-driven world, businesses and organizations are constantly seeking ways to gain a competitive advantage, make informed decisions, and shape their future strategies. This pursuit has given rise to the dynamic field of predictive analytics, a discipline that leverages historical and transactional data to predict future events, trends, and outcomes. At its core, predictive analytics is about using advanced data analytics techniques, including predictive modelling, data mining, machine learning, and neural networks, to analyze data and forecast future trends. In this article, we will delve into the world of predictive analytics, exploring how it transforms past data into actionable insights that can drive business decisions and strategies. We will also discuss the vital role that machine learning plays in enhancing the predictive capabilities of these models.
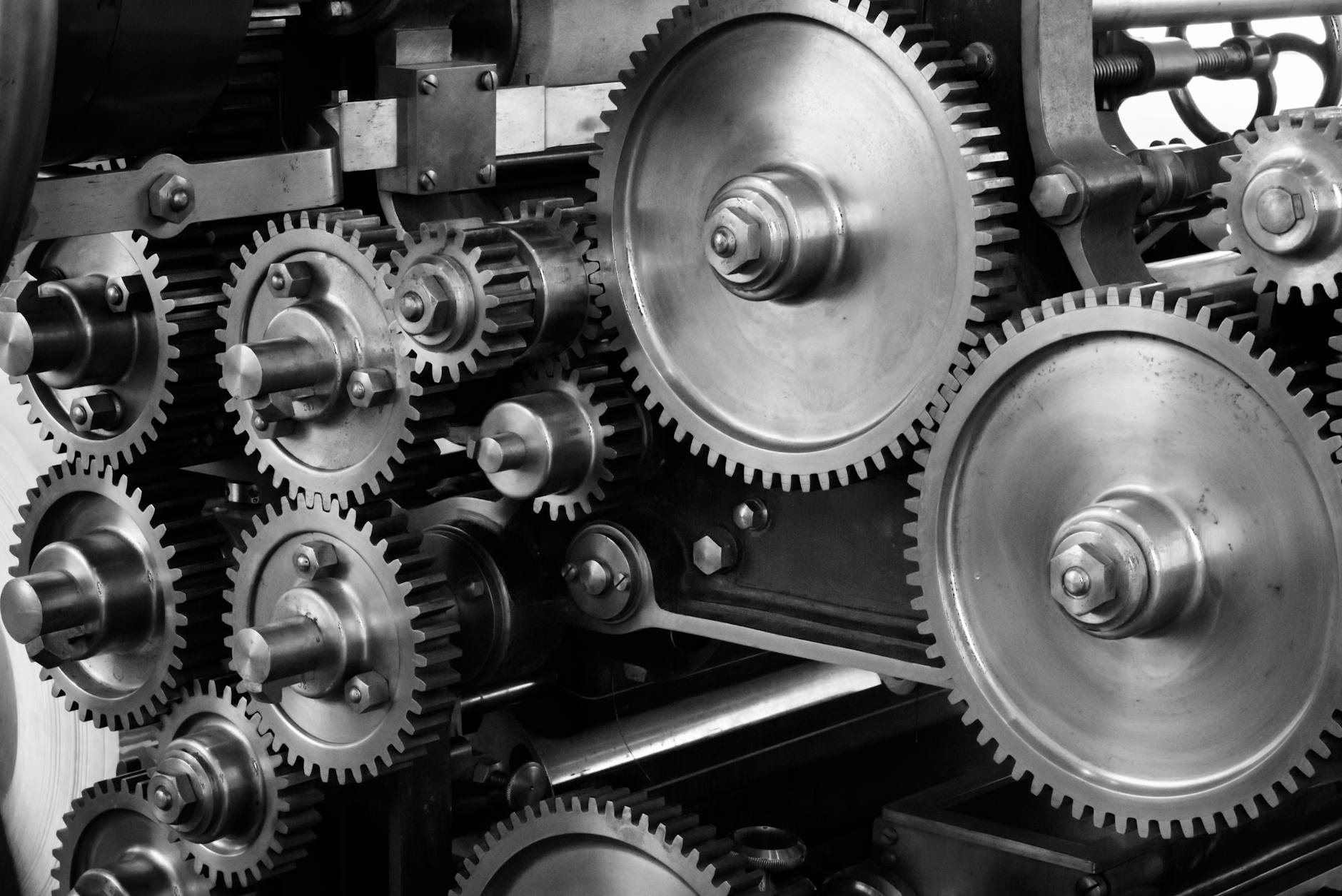
Understanding Predictive Analytics
Predictive analytics is a technological discipline that excels in forecasting future unknowns by harnessing various techniques, including artificial intelligence (AI), data mining, machine learning, modelling, and statistical methods. Predictive models find application in diverse fields such as weather forecasting, video game development, speech-to-text translation, and customer service enhancement. These applications rely on descriptive statistical models derived from historical data to make informed predictions about future data. Moreover, businesses find predictive analytics invaluable for inventory management, crafting effective marketing strategies, and predicting sales. In fiercely competitive industries like healthcare and retail, it becomes a lifeline, enabling organizations to not just thrive but survive. Furthermore, investors and financial professionals leverage this technology to construct investment portfolios and mitigate potential risks.
The Role of Machine Learning in Predictive Analytics
Machine learning, a subset of artificial intelligence (AI), is the engine that drives the predictive analytics revolution. At its essence, machine learning enables computer systems to learn from data and improve their performance over time without being explicitly programmed. This capability is crucial for predictive analytics, as it allows for the creation of models that can adapt and refine their predictions based on new data.
Key Trends in Machine Learning for Predictive Analytics
- Automated Machine Learning (AutoML)
- AutoML simplifies the process of building machine learning models by automating the selection of algorithms, feature engineering, and hyperparameter tuning. This trend is making predictive analytics more accessible to non-experts and accelerating the deployment of machine learning solutions.
- Explainable AI (XAI)
- As machine learning models become more complex, the need for transparency and interpretability grows. Explainable AI aims to make the decision-making process of machine learning models understandable to humans, which is crucial for gaining trust and ensuring compliance with regulations.
- Real-Time Predictive Analytics
- The demand for real-time insights is driving the integration of machine learning with streaming data platforms. This trend enables businesses to make immediate decisions based on the latest data, enhancing responsiveness and agility.
- Edge Computing
- With the proliferation of IoT devices, edge computing is becoming essential for predictive analytics. By processing data closer to the source, edge computing reduces latency and bandwidth usage, making real-time predictions more feasible.
Case Studies in Machine Learning for Predictive Analytics
- Healthcare: Predicting Patient Outcomes
- Machine learning models are being used to predict patient outcomes, such as the likelihood of readmission or the progression of diseases. For example, predictive analytics has been employed to forecast the spread of infectious diseases, enabling healthcare providers to allocate resources more effectively.
- Retail: Demand Forecasting
- Retailers use predictive analytics to forecast demand for products, optimize inventory levels, and reduce stockouts. Machine learning models analyze historical sales data, seasonal trends, and external factors to provide accurate demand predictions.
- Finance: Fraud Detection
- Financial institutions leverage machine learning to detect fraudulent transactions in real time. Predictive models analyze transaction patterns and flag anomalies that may indicate fraud, helping to prevent financial losses.
- Manufacturing: Predictive Maintenance
- In manufacturing, predictive analytics is used to predict equipment failures before they occur. Machine learning models analyze sensor data from machinery to identify patterns that precede breakdowns, allowing for timely maintenance and reducing downtime.
Conclusion
Machine learning is revolutionizing predictive analytics by enhancing the accuracy and efficiency of predictions. As businesses continue to generate vast amounts of data, the importance of predictive analytics will only grow. By staying updated with the latest trends and leveraging machine learning, organizations can unlock valuable insights and drive strategic decision-making. Whether it’s predicting patient outcomes in healthcare, forecasting demand in retail, detecting fraud in finance, or preventing equipment failures in manufacturing, the applications of machine learning in predictive analytics are vast and transformative.